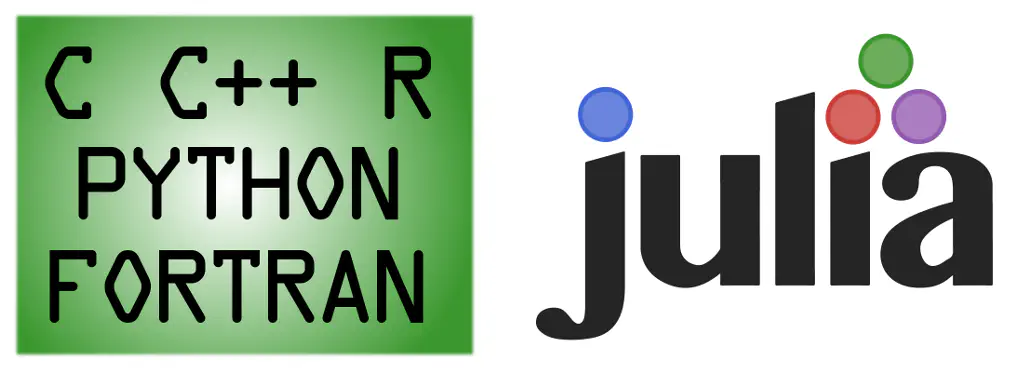
Julia has native support for calling C and FORTRAN functions. There are also add on packages which provide interfaces to C++, R and Python. We’ll have a brief look at the support for C and R here. Further details on these and the other supported languages can be found on github.
Why would you want to call other languages from within Julia? Here are a couple of reasons:
- to access functionality which is not implemented in Julia;
- to exploit some efficiency associated with another language.
The second reason should apply relatively seldom because, as we saw some time ago, Julia provides performance which rivals native C or FORTRAN code.
C
C functions are called via ccall()
, where the name of the C function and the library it lives in are passed as a tuple in the first argument, followed by the return type of the function and the types of the function arguments, and finally the arguments themselves. It’s a bit klunky, but it works!
ccall((:sqrt, "libm"), Float64, (Float64,), 64.0)
8.0
It makes sense to wrap a call like that in a native Julia function.
csqrt(x) = ccall((:sqrt, "libm"), Float64, (Float64,), x);
csqrt(64.0)
8.0
This function will not be vectorised by default (just try call csqrt()
on a vector!), but it’s a simple matter to produce a vectorised version using the @vectorize_1arg
macro.
@vectorize_1arg Real csqrt;
methods(csqrt)
# 4 methods for generic function "csqrt":
csqrt{T<:Real}(::AbstractArray{T<:Real,1}) at operators.jl:359
csqrt{T<:Real}(::AbstractArray{T<:Real,2}) at operators.jl:360
csqrt{T<:Real}(::AbstractArray{T<:Real,N}) at operators.jl:362
csqrt(x) at none:6
Note that a few extra specialised methods have been introduced and now calling csqrt()
on a vector works perfectly.
csqrt([1, 4, 9, 16])
4-element Array{Float64,1}:
1.0
2.0
3.0
4.0
R
I’ll freely admit that I don’t dabble in C too often these days. R, on the other hand, is a daily workhorse. So being able to import R functionality into Julia is very appealing. The first thing that we need to do is load up a few packages, the most important of which is RCall
. There’s great documentation for the package here.
using RCall
using DataArrays, DataFrames
We immediately have access to R’s builtin data sets and we can display them using rprint()
.
rprint(:HairEyeColor)
, , Sex = Male
Eye
Hair Brown Blue Hazel Green
Black 32 11 10 3
Brown 53 50 25 15
Red 10 10 7 7
Blond 3 30 5 8
, , Sex = Female
Eye
Hair Brown Blue Hazel Green
Black 36 9 5 2
Brown 66 34 29 14
Red 16 7 7 7
Blond 4 64 5 8
We can also copy those data across from R to Julia.
airquality = DataFrame(:airquality);
head(airquality)
6x6 DataFrame
| Row | Ozone | Solar.R | Wind | Temp | Month | Day |
|-----|-------|---------|------|------|-------|-----|
| 1 | 41 | 190 | 7.4 | 67 | 5 | 1 |
| 2 | 36 | 118 | 8.0 | 72 | 5 | 2 |
| 3 | 12 | 149 | 12.6 | 74 | 5 | 3 |
| 4 | 18 | 313 | 11.5 | 62 | 5 | 4 |
| 5 | NA | NA | 14.3 | 56 | 5 | 5 |
| 6 | 28 | NA | 14.9 | 66 | 5 | 6 |
rcopy()
provides a high-level interface to function calls in R.
rcopy("runif(3)")
3-element Array{Float64,1}:
0.752226
0.683104
0.290194
However, for some complex objects there is no simple way to translate between R and Julia, and in these cases rcopy()
fails. We can see in the case below that the object of class lm
returned by lm()
does not diffuse intact across the R-Julia membrane.
"fit <- lm(bwt ~ ., data = MASS::birthwt)" |> rcopy
ERROR: `rcopy` has no method matching rcopy(::LangSxp)
in rcopy at no file
in map_to! at abstractarray.jl:1311
in map_to! at abstractarray.jl:1320
in map at abstractarray.jl:1331
in rcopy at /home/colliera/.julia/v0.3/RCall/src/sexp.jl:131
in rcopy at /home/colliera/.julia/v0.3/RCall/src/iface.jl:35
in |> at operators.jl:178
But the call to lm()
was successful and we can still look at the results.
rprint(:fit)
Call:
lm(formula = bwt ~ ., data = MASS::birthwt)
Coefficients:
(Intercept) low age lwt race
3612.51 -1131.22 -6.25 1.05 -100.90
smoke ptl ht ui ftv
-174.12 81.34 -181.95 -336.78 -7.58
You can use R to generate plots with either the base functionality or that provided by libraries like ggplot2 or lattice.
reval("plot(1:10)"); # Will pop up a graphics window...
reval("library(ggplot2)");
rprint("ggplot(MASS::birthwt, aes(x = age, y = bwt)) + geom_point() + theme_classic()")
reval("dev.off()") # ... and close the window.
Watch the videos below for some other perspectives on multi-language programming with Julia. Also check out the complete code for today (including examples with C++, FORTRAN and Python) on github.